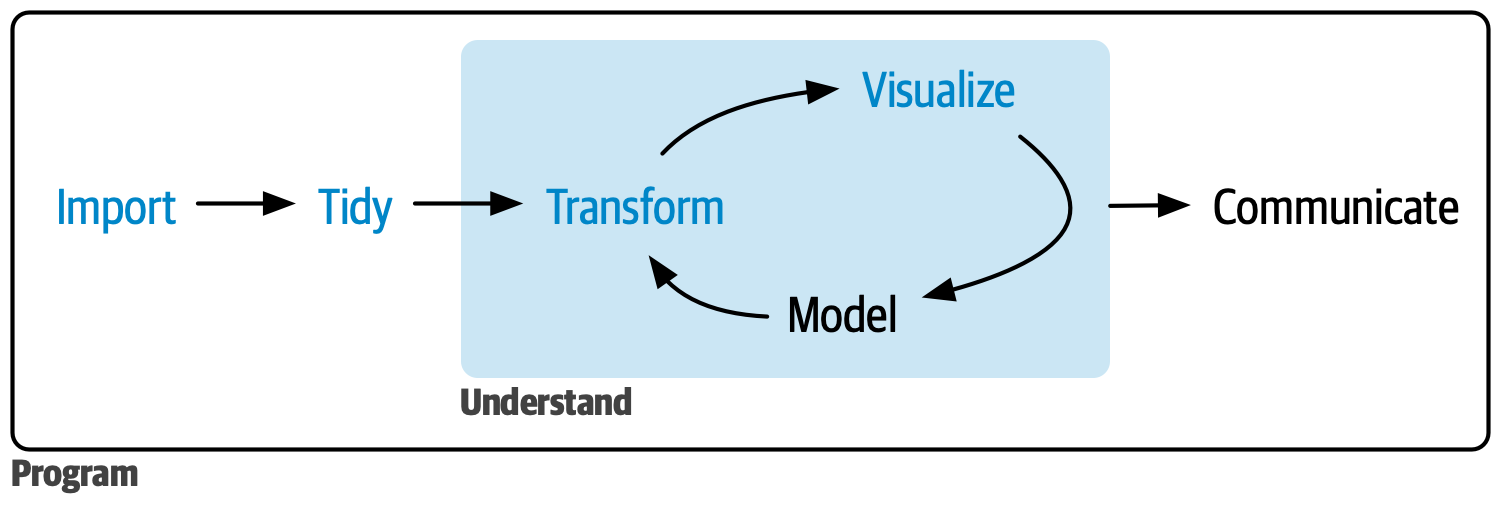
Whole game
Our goal in this part of the book is to give you a rapid overview of the main tools of data science: importing, tidying, transforming, and visualizing data, as shown in Figure 1. We want to show you the “whole game” of data science giving you just enough of all the major pieces so that you can tackle real, if simple, datasets. The later parts of the book will hit each of these topics in more depth, increasing the range of data science challenges that you can tackle.
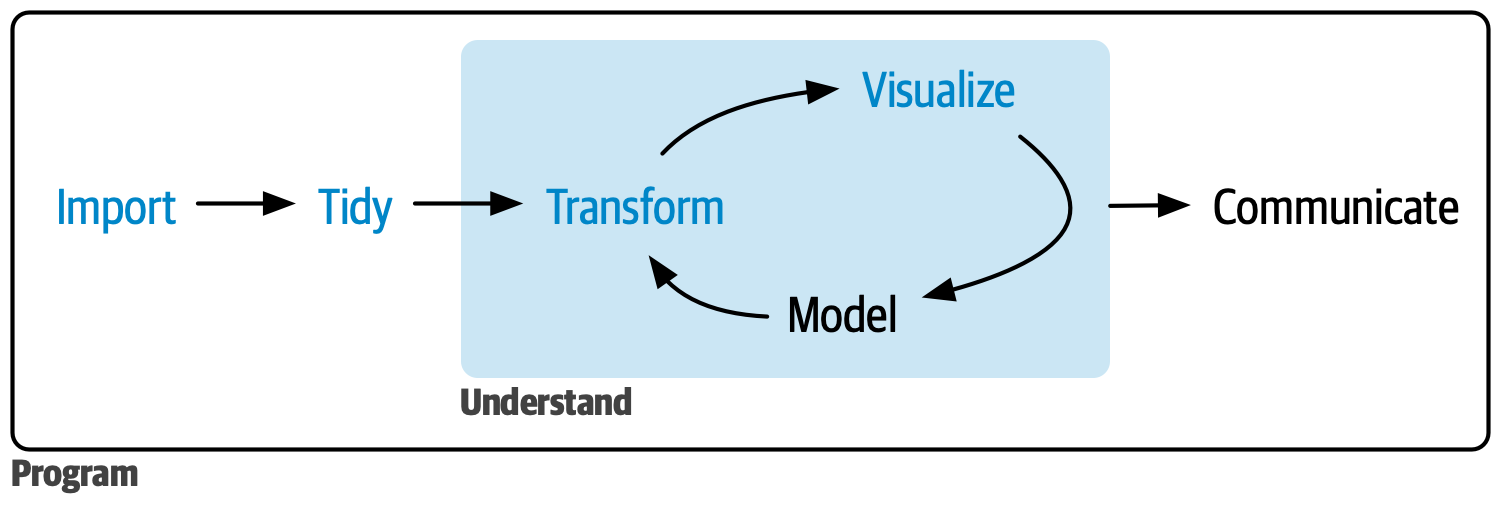
Four chapters focus on the tools of data science:
Visualization is a great place to start with R programming, because the payoff is so clear: you get to make elegant and informative plots that help you understand data. In 1 Data visualization you’ll dive into visualization, learning the basic structure of a ggplot2 plot, and powerful techniques for turning data into plots.
Visualization alone is typically not enough, so in 3 Data transformation, you’ll learn the key verbs that allow you to select important variables, filter out key observations, create new variables, and compute summaries.
In 5 Data tidying, you’ll learn about tidy data, a consistent way of storing your data that makes transformation, visualization, and modelling easier. You’ll learn the underlying principles, and how to get your data into a tidy form.
Before you can transform and visualize your data, you need to first get your data into R. In 7 Data import you’ll learn the basics of getting
.csv
files into R.
Nestled among these chapters are four other chapters that focus on your R workflow. In 2 Workflow: basics, 4 Workflow: code style, and 6 Workflow: scripts and projects you’ll learn good workflow practices for writing and organizing your R code. These will set you up for success in the long run, as they’ll give you the tools to stay organized when you tackle real projects. Finally, 8 Workflow: getting help will teach you how to get help and keep learning.